More than melodies: Ethics of generative AI for music [Unfair use? series, Part 3] 🗣️
Overview of 10 more ways other than creating melodies that AI (specifically, generative AI) is being used in creation of music, with comments on ethicality and the 3Cs. (Audio; 12:41)
This post covers 10 ways (in addition to creating and performing melodies) that AI is being used in creation of music. It is a bonus in our 8-part series on ethics of generative AI for music, announced in this INTRODUCTION post on . Subscribe to be notified when new articles are published (it’s FREE!)
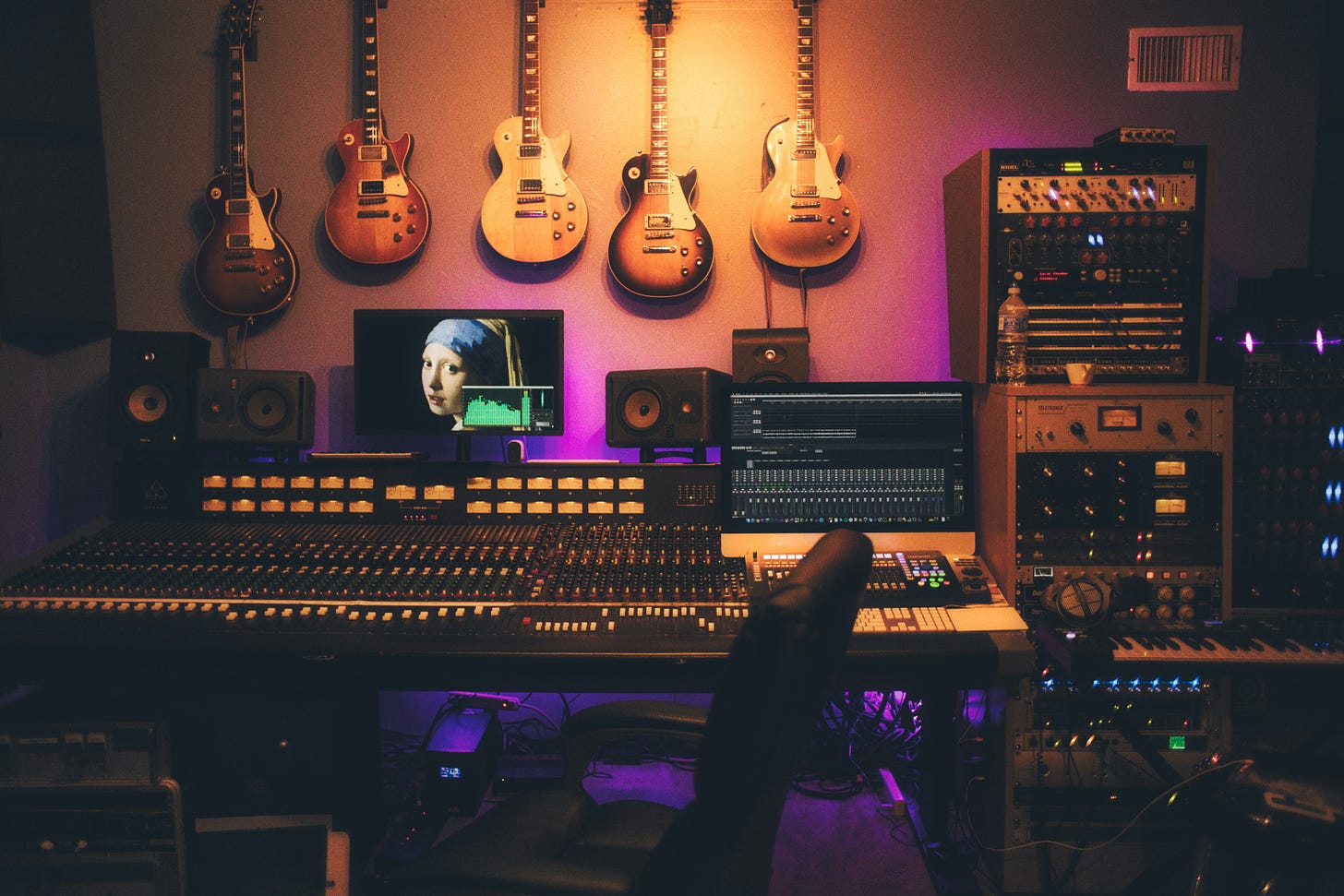
This article is not a substitute for legal advice and is meant for general information only.
More Than Melodies: other aspects of generating music with AI
In our article series on ethics of generative AI for music, we’re analyzing genAI tools for creating (composing and performing) melodies with instruments and/or vocals. However, melodies are clearly not the only aspects of music that can be manipulated or generated by AI. While working on Part 3 of our series, we’ve uncovered relevant tools and links for 10 other aspects. We are sharing them on this page for reference, in case they are of use to others.
1. Automated mastering
Mastering is “the final step of audio post-production” and is “done using tools like equalization, compression, limiting and stereo enhancement”. The goal of mastering is “to ensure your audio will sound the best it can on all platforms”. 1 AI-based mastering can automatically detect the genre and style and determine how best to adjust the loudness, highs and lows, and other aspects.
“Mastering is the process where an audio engineer takes your mix and zhuzhes it up, making everything match, sound right, get the best balance, and sometimes even pull more from the music than the original composer was able to mix in originally.” 2
LANDR is one example of an AI-based automated mastering tool. They launched in Canada in 2014 and bill themselves as “the creative platform for musicians: AI-powered music mastering, distribution, plugins, collaboration, promotion and sample packs”. 3
Ethicality of AI-based automated mastering tools depends on whether:
Whether remixing a song one does not own (for instance, to slow it down or speed it up) is ‘fair use’, vs. illegally creating a derivative work, has been a disputed area of the law.
A creator who owns the song and is mastering it with AI is clearly within their rights. Their use is likely to be ethical (provided the base model used in the tool was fairly trained).
2. Automated translation
AI can help with translating sung lyrics and vocals to new natural languages, for international audiences. As one example: Musician Lauv is working with AI voice startup Hooky to translate one of his songs into Korean.6
Ethicality of AI-based automated translation tools depends on whether:
the base model used in the tool was ‘fairly trained’, and
the owner of the rights to the original recording has consented, been credited, and is being properly compensated (3Cs).
It’s worth noting that automated translation for music has the potential for inadvertently creating an offensive rendition in the new language. This is an easy-to-overlook aspect of ethicality for auto-translating music lyrics with AI, just as it is for translation of texts. Review of the translated song by a native speaker of the new language can mitigate this risk.
3. Enhanced auto-tune
Auto-Tune was introduced in 1997 based on auto-correlation (signal processing, i.e. a non-AI feature). It was pioneered by Cher in her 1998 song “Believe” and became a signature sound for T-Pain and other artists.7 The technology has progressed to the point where some artists now use real-time Auto-Tune in a live concert, not just in recording a song or album. 8 Although Auto-Tune originated without AI, universities are currently experimenting with improving Auto-Tune with AI. 9
Ethicality of AI-based auto-tuning tools depends primarily on whether the base model used in the tool was ‘fairly trained’.
4. Functional music
Functional music is the term for individualized melodies created for therapeutic or wellness purposes. As mentioned in Part 1, “AI can be used to generate personalized tracks, adjusted to each person’s individual emotions and needs to create a therapeutic experience. It can generate calming melodies for anxiety relief, or upbeat tracks for motivation. It can even potentially analyze your reactions, preferences, and current behavior to fine-tune the music in real-time.” 10
Amazon’s Feb. 2023 “playlist partnership” with Berlin-based startup Endel addresses this use case. 11 Other therapeutic applications, such as “music-based reminiscence” to support mental health of older adults, are also being explored.12
Provided the underlying model has been ‘fairly trained’, functional music appears to be one of the more ethical uses of generative AI for music.
5. Generating lyrics
Large language models are trained on massive datasets with words, and associated metadata. Just like they can be used to generate emails or articles or customer support responses, they can be used to generate lyrics.
Ethical considerations of genAI for creating lyrics are the same as for other text. The music contributors who own the lyrics used to train the underlying models should have the opportunity to consent, be credited, and be properly compensated (3Cs).
6. Music discovery / recommenders / playlist generators
Music discovery tools help you find more music you may like. They may be recommenders for a single song, an “AI DJ”, or a playlist generator.
Some examples: Spotify “AI DJ” and “AI Playlist” 13, Amazon Music “Maestro” 14, Harmix 15, Chosic "similar song finder" 16, Song Hunt 17, deepAI 18, CLaMP 19 (this is not an exhaustive list).
Ethicality of music discovery tools depends on whether the base model used in the tool was ‘fairly trained’.
7. Self-organizing sample managers
A sample manager can help a music creator or producer to organize and leverage their library of samples by enabling them to tag (and then search by) key, bpm, instrument, genre, mood, and more. 20
On the surface, tools like Splice which use “AI” seem to only use machine learning for searching, not for generating music. Another sample manager, Cosmos Sample Finder, “utilizes artificial intelligence to manage and auto-tag your entire sample library” - but it’s not clear if it’s really AI, or even generative AI.
Ethicality of sample management tools depends on whether the base models used in the tool for auto-tagging or search were ‘fairly trained’. For search, it’s probably not much different from other search tools for other modes of media.
8. Stem splitting
Stem splitting means dividing an audio recording into separate parts for each instrument, including individual voices. 21 Some examples: Audioshake, LALAL.AI, Musicfy AI Karaoke Maker 22 and AI Stem Splitter 23.
Stem splitting is typically used to generate “karaoke tracks” and as one step in tools for generating vocal covers (below). Stem splitting with generative AI separates and removes the original voice (or an original instrument) from a recording. This enables the tool to substitute the user’s voice or instrument on top of the original instruments.
Ethicality of stem splitting depends on whether:
the base model used for the splitting was ‘fairly trained’, and
the owner of the rights to the original recording has consented, been credited, and is being properly compensated (3Cs).
9. Voice cloning / synthesis
Voice cloning is creating a unique genAI model for a single person’s voice, using recordings of that person’s voice plus a foundation model trained on many people’s voices. (‘Voices’ of played musical instruments can be similarly cloned.)
See bonus article #1 for why the current VC tools are (mostly) unethical IMHO, especially when the voice is not one’s own.
See bonus article #2 for details on non-music text-to-speech voice cloning, and the few ethical tool providers we’ve identified.
Voice synthesis uses a voice clone model to generate ‘new’ music. In practice, voice synthesis is sometimes conflated with voice cloning. A tool that can create a voice clone model can typically also use it for voice synthesis.
As an example use of voice synthesis: A composer might use a tool with a pretrained, canned voice clone model to ‘sing’ their lyrics and quickly create a ‘low-fidelity prototype’ of their song, for pitching it to producers and performers. Provided the pretrained voice clone model was ethically acquired, and applied to the composer’s own song, this could be an ethical use.
Some other example uses of voice synthesis:
for making new “tribute” recordings of deceased singers
for record labels to record promotions of a deceased singer’s works “in their own voice” (speech or singing) for advertising purposes
Ethicality of voice synthesis will vary greatly depending on whether:
the base model used for the synthesis was ‘fairly trained’, and
the owners of the rights to the original voice have consented, been credited, and are being properly compensated (3Cs).
10. Vocal cover
A “cover” of a song is when a musician substitutes their own performance for part or all of the performance by the original artist.24 Karaoke is essentially a vocal cover assistant: a karaoke track has the original voice removed (by stem splitting) so that you can sing along.
AI-based vocal cover tools use ‘style transfer’ to apply a voice clone model trained on a new voice to a song that was recorded by a different performer. (Like with a sung vocal, musical instruments can be substituted or applied from a ‘voice’ clone.) These tools don’t generate new melodies; they generate new recordings having a substitute track with the new voice or instrument.
Ethicality of vocal covers depends on whether:
the base model used for voice cloning and synthesis was ‘fairly trained’, and
the owners of the rights to the original recording have consented, been credited, and are being properly compensated (3Cs).
We do not plan to cover voice cloning, vocal cover, or voice synthesis features further in this series.
IP Rights of Stakeholders
In the sections above, we refer to “the owners of the rights”. Who are these owners? Well, in PART 1 of our series, we identified 3 groups of stakeholders: music contributors, music users, and tool providers. Music contributors include composers, performers, and production companies. One or more of them are generally the owners of the rights.
More than one kind of IP rights are relevant for music contributors. They can be classified as Composition rights, Master rights, and Performer Rights. If you’re interested in a deeper understanding of these kinds of IP rights and how they apply to various music contributors, check out this excellent Bandlab article 25 (discovered in April after Part 1 was published) or this description of music copyrights from SoundCharts 26.
What’s Next?
GenAI tools in the above categories are outside our focus in this article series. However, it’s worth noting that many of the same companies that are using genAI for melodies are also using it for some of the purposes listed above (e.g. vocal covers - example: ElevenLabs). The offerings from these companies will be discussed in varying depth in PART 3.
This page will be updated from time to time, as new tools and applications come out. Feel free to comment or link with any suggestions or additions!
References
See this “AI for Music” page for a complete set of links to all posts on AI for music.
End Notes
“What is mastering?” by LANDR, undated (retrieved 2024-05-13)
“How AI helped get my music on all the major streaming services”, by ZDnet / David Gewirtz, 2023-08-10
Notes on LANDR:
This article says that the tool “basically gets new data from every track that's uploaded to it, analyzes it and learns from it” (ref: “LANDR Technology Interview”, Vice / Greg Bouchard, 2014-08-03)
It “decides on basic genre detection that informs an overall mastering style. It responds uniquely, it never does the same thing twice, and the beauty of it is that really learns from user reactions.” (ref: “Meet LANDR: A Service That Masters Your Tracks Instantly”, Vice / Jemayel Khawaja, 2014-07-23)
They announced an “AI-based plugin” in Oct. 2023, touting that it is “trusted by more than 5M artists, mastering over 25M songs in the past decade” (ref: “LANDR launches new AI-powered mastering plugin for digital audio workstations”, AIthority / By PRNewswire, 2023-10-20).
In brief, models that have been ‘fairly trained’ must grant the 3Cs (consent, credit, and compensation [5]) to all providers of data used in training the models. Startup Fairly Trained offers certification of models which meet their criteria. Certification is a good step, but lack of certification does not mean a tool is unethical or was unfairly trained. It just means you need to do your own homework to try to determine if the tool is ethical or not.
“What an anonymous artist taught us about the future of AI in music”, MSN / Kristin Robinson, 2023-12-11
Auto-Tune ref: Musicfy post
References for use of auto-tune in live performances:
Research into AI for auto-tune - Johns Hopkins ref
“AI in music: generative music, deepfakes, and more”, Hype Magazine, by Jerry Doby, Aug. 2023
“Exploring the Design of Generative AI in Supporting Music-based Reminiscence for Older Adults”, Yucheng Jin,Wanling Cai, Li Chen, Yizhe Zhang, Gavin Doherty, and Tonglin Jiang. Proceedings of the CHI Conference on Human Factors in Computing Systems (CHI ’24), May 11–16, 2024, Honolulu, HI, USA. ACM, New York, NY, USA, 17 pages. https://doi.org/10.1145/3613904.3642800
Spotify music discovery links:
rolled out an “AI DJ feature” in 2023
just released an “AI playlist” creator as beta for UK and Australia in April 2024
“Spotify releases generative AI playlist creator”, voicebot.ai, 2024-04-08
“Spotify now lets you create an AI-made playlist with just a text prompt, ZDnet, 2024-04-08
Amazon Maestro links:
launched on April 16 as beta (in both the iOS and Android mobile apps)
“Amazon Music echos Spotify with an AI playlist generator of its own”, ZDnet, April 16, 2024: “On Tuesday, Amazon Music launched Maestro, an AI playlist generator that responds to a user's text, emoji, or voice prompt to create a new playlist with a unique selection of tracks”
Harmix links:
No info on source of its 2.1m songs in “Discover the future of music search: Introducing Harmix’s groundbreaking AI service”, musically, 2024-01-18) or harmix.ai/terms-of-use - they refer to Licensors but do not specify
Other sites, e.g. https://www.aimusicpreneur.com/ai-tools/harmix/, refer to searching a user’s catalogs and not Harmix’s catalog (?)
Chosic “similar song finder”: https://www.chosic.com/playlist-generator/
deepAI music discovery: https://deepai.org/chat/songs
Microsoft Muzic CLaMP “Similar Music Recommendation - a Hugging Face Space by sander-wood”
“How to Use a Sample Manager To Organize Your Sample Library”, audiocipher / Ezra Sandzer-Bell, 2023-07-15 - article describes purpose of sample managers and overviews 6 sample management tools
“The Best Music-Making AI Tools and How to Use Them”, Resident Advisor / guest-edited by Cherie Hu, June 2023
“Music Rights: Insights and Implications”, BandLab blog, undated (retrieved 2024-05-13)
“6 Basics of Music Copyright Law: What It Protects and How to Copyright a Song”, SoundCharts Team, December 31, 2023