4 pillars of ethical genAI for music [Unfair use? series, Part 2] 🗣️
Clarifying the scope of our series on ethics of generative AI for music, and sharing the 4 pillars of our framework for analyzing ethics to cover the 4Cs (audio now available; 16:52)
The war saying “No plan survives first contact with the enemy”1 is also true in writing about topics as dynamic as AI today! This article shares how our plan for this series on ethics of generative AI has evolved since March. It elaborates on the 4 pillars of our framework for analyzing ethics of AI companies and maps the pillars to the “4Cs” (consent, control, credit, and compensation).
This post is part of our series on ethics of generative AI for music in . Subscribe to be notified automatically when new articles are published (it’s FREE!)
This article is not a substitute for legal advice and is meant for general information only.
Post Contents:
Acronyms
4Cs - Consent, Control, Credit, and Compensation to contributing artists (for use of their content) 2
QDPs - companies with Questionable Data Practices (term defined by Ed Newton-Rex in his May 22 Substack article on Suno)
AI Ethics in Music
The field of competitors offering products using generative AI to create musical melodies is vast and growing. Our goal is to help shed light on the ethical positions and risks of these companies and their products. For some, their AI tactics clearly show multiple bright red flags. For others, we just don’t know enough yet. We’re on a mission to find out and share the info with you, so we can all make better-informed decisions.
What’s ETHICAL in use of generative AI for music? At a minimum: avoiding use of unlicensed content (music and voices) and ensuring the “4Cs” (consent / control, credit, and compensation [1]) for contributing artists. (See PART 2 and below for more specifics.)
What “Fairly Trained” Means
Resistance to exploitative genAI tools has gained traction in the past 6+ months 3 4. Example: Ed Newton-Rex, inventor of Jukedeck and lead creator of Stable Audio, quit Stability AI in November 2023 in protest over what he saw as exploitative use of unlicensed content for their image AI product 5. He now leads a startup, “Fairly Trained”, focused on verifying whether a model underlying a genAI product has been developed ethically and avoids QDPs (Questionable Data Practices) 6.
As we noted in our May 23 article, complementary approaches to Fairly Trained are now emerging for verifying ethicality of AI-generated music. Example: the May 21 announcement by Voice-Swap and BMAT (“the operating system for the music industry”) on a partnership to use technology to verify music sources.
It’s clearly possible that some companies who are operating ethically have not yet undertaken or completed a certification or verification process. We discuss this in our next article (PART 3) where we summarize 85 companies working on generative AI for music.
Four Pillars of AI Music Ethics
As we identified in PART 2, how the underlying model was developed is not the only consideration in whether a genAI-based product is ethical. We are looking at 4 pillars. Each pillar will be the focus of a deep dive in PARTs 4-7.
Pillar 1: Training & Reference Data [PART 4]
Generative AI models, and many machine learning models, rely on a large base of source data for training a foundational model. Whether an AI or ML model is fairly trained with ethically-sourced data covers part of this pillar, but not all of it.
If a tool allows users to supply their own song snippets, stems, voices, or reference URLs, ethics require precautions to ensure that these uses are authorized.
In either case, ethical tools must ensure that the 4 Cs [1] are covered for the music contributors for the training data or reference audio. Contributors deserve the opportunity for meaningful consent; to have control over how their work is used; to be credited when their work is used; and to be compensated fairly for it.
The 4 Cs also apply in principle to music users who use these tools to generate new tracks. Ethical tool providers who use their outputs to help train their tools should offer their users the opportunity to consent to use of their work. Ideally, this would be through opt-in. If it’s handled through the terms and conditions of use of the tool, ethicality requires that they be highly transparent with their users that they are waiving their rights to the 4Cs for their creations in exchange for use of the tool.
Pillar 2: Traceability & Fair Use [PART 5]
Traceability and fair use include:
transparent labeling on whether a track was created with AI (and if so, which tool), plus
data provenance so that artists, tool users, and even listeners can know whose source music went into an AI-generated track.
Traceability is a mandatory “enabler” for achieving the 4Cs. Contributors cannot be properly credited and compensated without traceability to where their content is used.
Pillar 3: Bias Minimization [PART 6]
Minimizing bias requires proactive consideration of possible biases in the source datasets, for:
scrubbing incoming data used for training models to ensure accuracy and validity and to identify sources of bias,
ensuring that creeping or injected biases can be prevented (ideally) or detected & mitigated quickly, and
ensuring equitable access of the tools (e.g. to disabled users7).
Detection and mitigation of biases and accessibility issues will always be needed. 100% prevention of bias is likely unachievable. That’s no excuse for not diligently addressing prevention as a high priority, or not designing up front for accessibility.
Pillar 4: Cost-Benefit Analysis [PART 7]
Ethicality requires weighing the benefits of AI use vs. the costs. Costs must include assessing environmental impact; training and running generative AI have a heavy ecological footprint.
Mapping the Four Pillars to the 4Cs
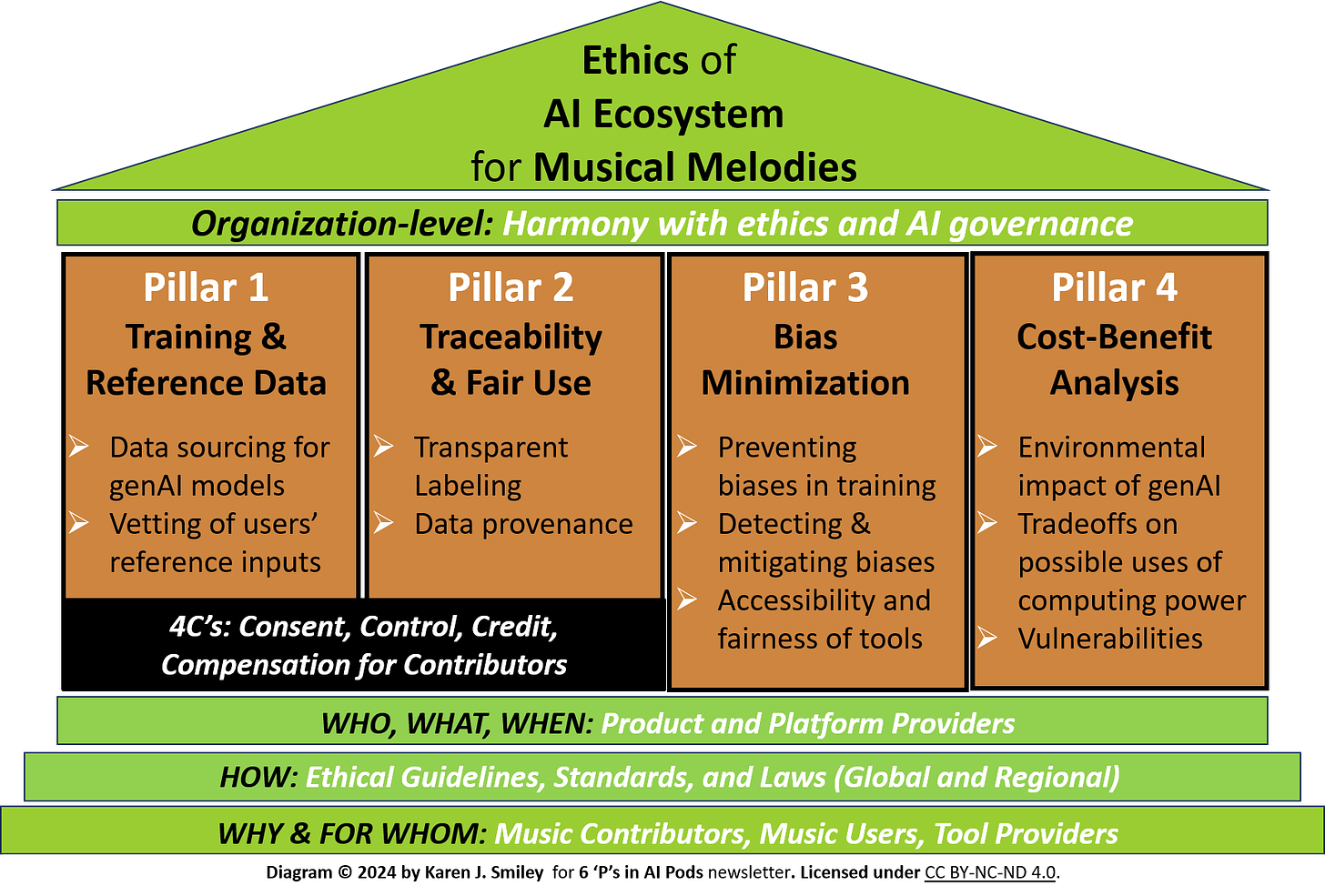
The first two pillars (training and reference data, traceability and fair use) span the 4Cs (consent, control, credit, and compensation) [1] for music contributors. Therefore, while fair training and support for the 4Cs are necessary for tools using generative AI models, they are not sufficient for an AI company or product in this space to be fully ethical: all four pillars must be covered.
In addition to Fairly Trained and Voice-Swap + BMAT, other startups are exploring areas relevant to AI ethics for music which align with the four pillars:
Traceability and licensing (example: Rightsify; their HYDRA music model is Fairly Trained)
Transparency about use of AI (examples: AI Voice Detector (link) and PlayHT (link)). (reference - MakeUseOf)
Bias checking tools: for more on this topic, see ’s post on frameworks supporting GDPR for AI.
For now, knowing if a company’s products are Fairly Trained is as good a starting point as any available to us. We use it in PART 3 for grouping and selecting companies to profile.
Scope Adjustments
Our “INTRO” post in mid-March set out our plan for this series on AI for Music. In the past 4 months, we’ve learned more about the topic. It’s clear that the ocean of generative AI for music is too big for just a few contributors to boil quickly.
We’ve evolved our plan for this series on ethics of AI for music. To keep our quest to a manageable size, we’ve clarified some boundaries and excluded some scope. Focusing closely on uses of AI for musical melodies will still cover the important companies and ethical concerns that impact all areas of music, and should continue to provide some insights even beyond music.
AI vs. GenAI in Music
As noted in the Introduction to this series, use of true transformer-based generative AI for creating music is relatively new. However, AI and non-AI creation of music has been around for many years. And some recently-announced “AI-based” tools aren’t actually using genAI. (Credit to for this section)
As one example, in 2020 a professor in India announced creating a software tool that used AI to generate Indian classical music. However, it was not generative AI as the term is used today; it was basically a rule-based expert system.
The tool did not use a transformer-based model trained on a large repository of songs. It does not have a database of ragas (songs) - instead, it “generates the required musical data following the rules”. The inventor’s system “coded the concepts and composition rules of Indian classical music to generate a 'bandish' (composition) every time the vadi (dominant) and samvadi (sub dominant) swaras are provided as inputs.” (ref: “New software can compose Indian classical music with help from AI”, India Times, Jan 04, 2020)
Other examples:
MIDI Melody Generator is a free tool from dopeloop.ai that is not based on a GPT or LLM. Its melodies are “procedurally generated”.
Audiocipher (a “word-to-MIDI” music generator) is also algorithmic. It uses “musical cryptograms”, not AI. Text provided by the user is translated letter by letter into music notes.
Significant ethical risks in the first two pillars attach to training a generative AI model on a vast repository of possibly-unlicensed songs and data. “AI” tools like these which don’t use generative AI or ML, or don’t rely on a base of unethically-sourced data, do not carry the same risks. However, ethical risk pillars 3 and 4 (bias minimization and cost-benefit analysis) may still apply.
Notable Exclusions
Exclusion 1. More Than Melodies: other aspects of generating music
Melodies are far from the only aspects of music that are potentially amenable to AI-based manipulation or generation. Ten (at least) other types of music tools use AI: automated mastering, automated translation, enhanced auto-tune, functional music, generating lyrics, music discovery, self-organizing sample managers, stem splitting, voice cloning/synthesis, and vocal covers. For details on these ten, with comments on ethicality, see our May 13 article “More Than Melodies”.
The last two do overlap somewhat with musical melodies. Our April 28 article “AI Ventriloquism: Send in the clones” already covered voice cloning and vocal covers. Our May 9 article “Say What? Adding audio voiceovers with AI, ethically” evaluated 3 likely-ethical voice cloning tools (ElevenLabs, Speechify, and Wondercraft.ai). (However, if you are mulling use of ElevenLabs for music generation, see our follow-up profile on ElevenLabs.)
We do not plan further articles on these 10 aspects of AI-generated music. This excludes in-depth analysis on Spotify’s use of AI for music recommendation and understanding, functional music companies like Brain.fm, or companies like Muzeek who are using AI to support logistics of music performances.
Exclusion 2. Musician-controlled initiatives
We assume ethicality for artist-controlled initiatives where they own the AI models they build of their own music and voices. This post on musician-led AI initiatives shares a diverse set of examples:
Voice cloning - Holly Herndon, Grimes, FKA Twigs.
Voice restoration - Randy Travis, Beanie Sigel.
Collaborations ‘with’ deceased musicians - Natalie Cole, Paul McCartney.
Songs ‘by’ deceased musicians - Kim Kwang-seok, Teresa Teng, Anita Mui.
Exclusion 3. Geographic coverage limitations
In-depth information about technology companies based in eastern Asia is typically lagging and/or scarce on English-language websites (or translations). However, given their activity in other AI areas, the safe assumption is that they are also making strong progress on AI-based TTA (Text To Audio). Chinese company Baidu is the largest AI patent holder in the world (ref), and 01.ai has been leading the way in multi-modal open source AI (ref). It would have been surprising to not find AI activity in China relating to music.
Four major companies from China, Korea, and Singapore are covered in our July 1 post “4 AI music companies in Asia”. Two have over 100 million users each. All are partnering with major music labels.
BandLab Technologies, in Singapore
ByteDance (TikTok parent), in China
HYBE/Supertone, in South Korea
Tencent Media Entertainment (TME), in China
The detailed profiles for each company (linked above) share what we were able to discover about these top 4. Since details on their ethics and training data are sparser, they won’t be covered further in PARTS 4-7.
What’s Next?
Because of the depth of information on each of the companies we profile, each profile is in a separate post, named for the company. I don’t want to overwhelm you all with too many emails about the company profile posts (15 so far, 16 in the works!). So I’m placing them in a separate section of this newsletter called “profiles”.
Current and future subscribers are not opted into profiles by default. Please subscribe to the profiles section if you’d like to be notified when company profiles are posted in the future!
In PART 3, we’ll identify 85 companies involved in generative AI for music. We are using the 4 pillars described here, and the 4Cs, to analyze 31 companies in depth. Seven of those companies will be the subjects of deeper analysis vs. the 4 pillars in PART 4 through PART 7. See you there!
REFERENCES
Credits
Thank you to Ezra Sandzer-Bell for his helpful explanation of musical cryptograms. Ezra’s articles on AI-based music tools are excellent (some are linked below). I highly recommend following him on the Audiocipher blog!
Articles and bonus pages in this 6 ‘P's in AI Pods series
See this “AI for Music” page for links to all posts related to this series, as well as bonus articles mentioned above on voice cloning and other music-related topics.
Specific articles, in the order they are referenced in this post:
End Notes
“No Plan Survives First Contact With the Enemy”, Quote Investigator, 2021-05-04
Credit for the 4 Cs (consent, control, credit, compensation) phrasing goes to the Algorithmic Justice League (led by Dr. Joy Buolamwini).
Credit for the original 3 Cs (consent, credit, and compensation) belongs to CIPRI (Cultural Intellectual Property Rights Initiative) for their “3Cs' Rule: Consent. Credit. Compensation©.”
“What An Anonymous Artist Taught Us About The Future Of AI In Music”, by Kristin Robinson / MSN, 2023-12-11
“From Billie Eilish to Stevie Wonder, hundreds of musicians condemn AI's 'assault on human creativity'”, by Artie Beaty / ZDnet, 2024-04-02
“This Tech Exec Quit His Job to Fight Generative AI's Original Sin”, by Kate Knibbs / Wired, 2024-01-17
“Why AI fairness conversations must include disabled people”, Eileen O’Grady / Harvard Gazette, 2024-04-03