Ethical Risks & Challenges in GenAI music [Unfair use? series, PART 2]
Part 2 on ethics of generative AI for music - how the 3 stakeholder groups in Part 1 are affected by ethical concerns (including model biases), and the “pro-choice” view of ownership rights
Welcome to the second article in our series on ethics of generative AI for music, and how it affects PRODUCTS and PEOPLE!
This article series is not a substitute for legal advice and is meant for general information only.
Here in PART 2, we tackle HOW each stakeholder group identified in PART 1 is affected by ethical concerns (including ownership rights and model biases) and summarize 3 recommendations for handling genAI for music ethically.
PART 3 will address WHO, WHAT & WHEN (products and companies). PARTS 4-8 will dive deeply into the ethical concerns on genAI for music for 5 major companies, summarize, and share some recommendations. Subscribe to be notified automatically when new articles in the series are published - this newsletter is free!
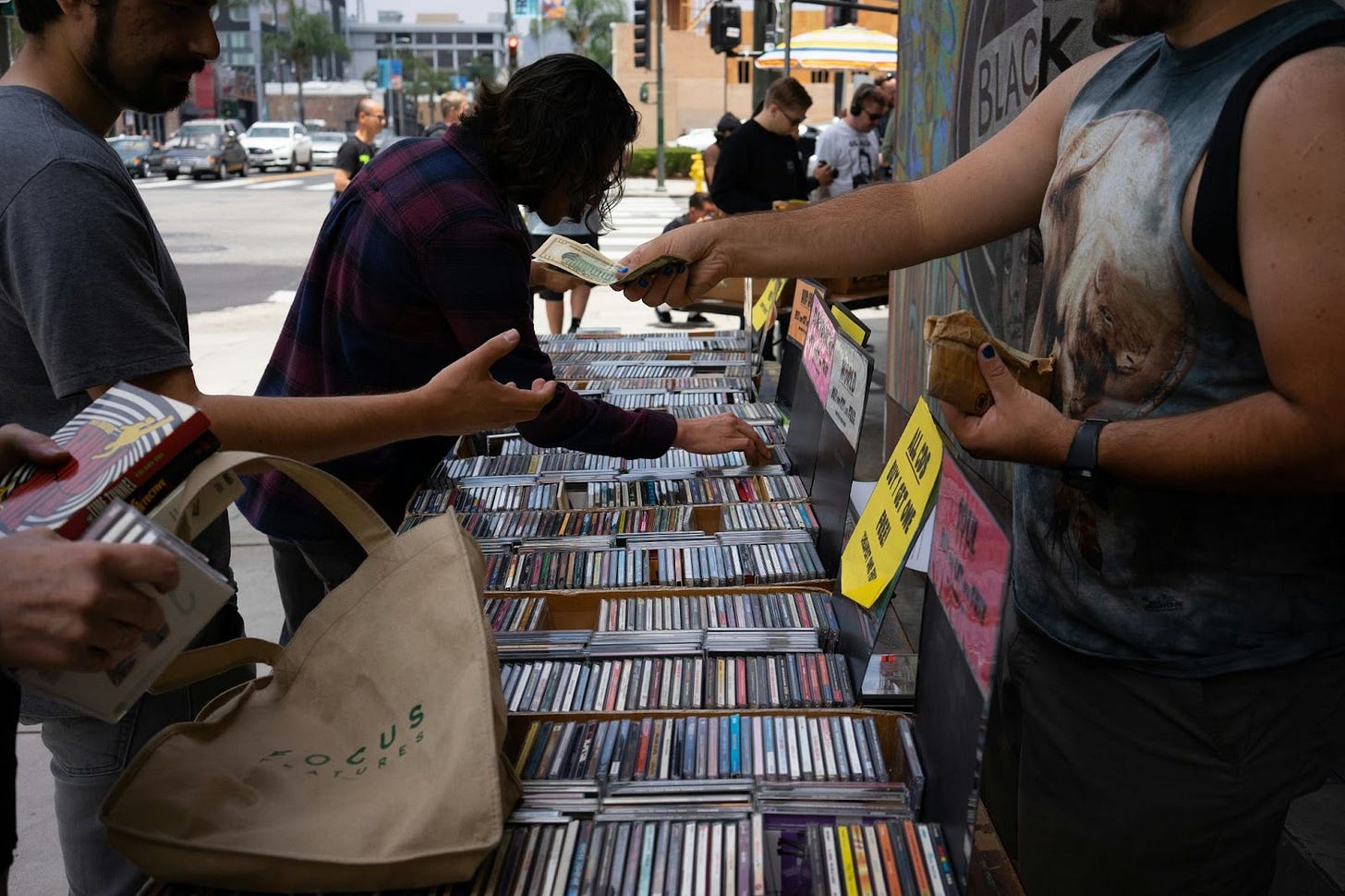
Table of Contents for this post: (Section headers will be made clickable after the article goes live - let me know if you find this TOC useful or not)
BACKGROUND on Key Ethical Risks and Challenges
HOW Music Composition Tools Are Using Generative AI
BACKGROUND on Key Ethical Risks & Challenges
The 3 stakeholder groups identified in PART 1 (music contributors, music consumers or tool users, and tool provider companies & staff) clearly have conflicting priorities. Livelihoods, reputations, and a great deal of money are at stake all around.
Decisions around uses of generative AI for creating music are further complicated “when considered against the environment in which they occur: a music industry dominated and directed by streaming platforms … [in] a complex socio-technical system that is reshaping music and our relationship with it”.1
How can we as a global society reconcile these priorities in a fair way? The short answer: AI ethics. In brief, ethics are how we decide between good and bad, or right and wrong.
“Ethics is inescapable because it concerns the ultimate values in which our choices are anchored, whether we realise it or not. These are values that define what it is to have a good life, and what we owe to others, including non-human animals and to nature. Therefore, all forms of ‘regulation’ that might be proposed for AI, whether one’s self-regulation in deciding whether to use a social robot to keep one’s aged mother company, or the content of the social and legal norms that should govern the use of such robots, ultimately implicate choices that reflect ethical judgments about salient values and their prioritisation.”2 - John Tasioulas, Ada Lovelace Institute
Debates on the ethics of AI began long ago - back in the 1960’s, according to this Oct. 2023 article “Navigating the Ethics of Artificial Intelligence”.3 The world is slowly (some say too slowly) making headway on defining regulations for ethical AI.
Do people today actually care about ethics of AI? A 2023 report by the Markkula Center for Applied Ethics at Santa Clara University on “Ethics in the Age of AI”4 concluded that:
82% of US-based respondents care about the ethics of AI, and 68% are somewhat or very concerned about the negative impact of AI on the human race
55% believe AI companies are not considering ethics when developing AI
“Who gets to use — and to commoditize — the art, text, and code created by others is now arguably the single biggest ethical question around AI” -
, in his March 15 post “The tide is turning against OpenAI”5So why is genAI for music ethically risky? And why are ethical solutions hard?
To understand how ethical concerns and guidelines apply to generative AI for music, we first need to understand how generative AI is being used for music.
HOW Music Composition Tools Are Using Generative AI
Aside from the use of generative AI to ‘create’ lyrics (and other use cases now described in “More Than Melodies”), there are (at least) two distinct ways generative AI is being used today in the creation of melodies or songs.
Generative AI to ‘compose’ melodies
The tools that are the focus of this article series all use generative AI for TTA (Text To Audio) to generate ‘new’ compositions. Google’s MusicGen tool can also take images as input. The Adobe blog states that, in addition to being able to put in a text prompt like “sad jazz” to generate music, “users could transform their generated audio based on a reference melody”, a capability MusicGen also supports.
Broadly speaking, there are two places in the tools where non-original content might be used to generate melodies or songs:
the foundational AI models, and
the reference melodies, snippets, or stems
Allowing a user to optionally supply a reference melody for “inspiration” is a bonus for musicians who want to build upon their own music. However, it seems to open the door to rampant copyright infringement by users who supply reference songs which they do not own, even if foundation models are trained solely on public domain or properly-licensed music.
AI-enhanced music editing
Adobe’s AI-based control features offer finer-grained editing support for extending the time, looping, etc. of a music clip.
From the perspectives of musicality, legal risks (infringement on copyrights of human-created music), and AI ethics, controlled editing features based on AI seem to create less risk of meaningful infringement of contributors’ rights than audio generation.
ETHICS & LEGALITY of Text-To-Audio (TTA) AI
As a lifelong music lover and career-long data and AI geek, I would love to try out all of these tools sometime soon. To date, I’ve been wary of unknowingly doing something unfair or unethical by using them.
And it’s not clear if these tools even produce good (or good enough) music that’s worth the effort and cost. (Creativity, ‘goodness’, and musicality of AI-generated songs, and tradeoffs of individual & societal costs vs. benefits for genAI music will be explored in PART 7 of this article series, Cost-Benefit Analysis)
Until recently, I didn’t have time to dig in and make sense of all of the noise and flashing lights around generative AI for music to figure out how I could use the tools ethically (and if that’s even possible today). I now have the time to prioritize this, so here goes 🙂
“Data authenticity, consent, and provenance for AI are all broken” - Shayne Longpre et al, March 276
“A review of AI research for this paper confirmed all known existing safeguards for LLMs are easily breached” - Mark Montgomery, 2023-2024 working paper7
The same ethical and legal questions that arise with generative AI for text, images, and video apply with using generative AI for audio and songs, although naturally the interpretations vary.
How do genAI applications impact the contributors whose copyrighted works are being fed into these tools, possibly without their consent or knowledge?
How carefully are these genAI tools being developed to ensure they will protect and be fair to their users, as well as to contributors?
What steps are being taken to measure and minimize bias creation or perpetuation?
Are the costs of using generative AI worth the benefits?
Specific to ethical use of generative AI for music, key questions about tools include:
Training and Reference Data: What songs are the models using?
What set of songs were the foundational models trained on? Were permissions or licenses obtained from the creators (songwriters and performers) for any non-public-domain songs used for training the models?
What set of songs is made available to a user for selecting a “reference melody”? If users can provide their own reference songs, how will legality be verified?
Traceability and Fair Use: How will tool providers ensure that fair use criteria are satisfied and that consumers (music users and listeners) can verify data provenance?
Bias Minimization: What precautions have been taken to ensure that the conversions of text prompts to audio content are as unbiased as possible, and that the models won’t learn inappropriate correlations of words to content and propagate newly biased content?
Cost-Benefit Analysis: Is use of AI appropriate? Both training AI models and using AI models are expensive operations (in computation resources & in corresponding environmental impact). Does the value gained by some stakeholders warrant the costs to society and the harms to other stakeholders?
Questions 1-4 can only be answered well per-tool and will be addressed in the deep dives (PARTS 4-7 in this series). For now, let’s cover some basic background on machine learning, AI biases, and ownership rights, and how they pertain to ethics of generative AI for music.
BIASES & ETHICS
Biases in machine learning algorithms are not new and can occur almost anywhere, even when developers try to avoid them. Almost any form of bias or discrimination you can name has already surfaced in a machine learning application in the real world and caused harm.
For example, in 2020, researchers discovered that Twitter’s automatic image cropping algorithm showed gender and racial biases8 (favoring white faces and women). Twitter later apologized9, noting that they had tested their cropping model for gender and racial biases prior to deployment, and didn’t find any, and have work to do.
If minimizing bias in AI/ML was easy, it would have already been done. But it doesn’t happen automagically; it takes work.
Why is it hard? Part of the answer lies in:
how machine learning and AI algorithms work, and
how we collect & use data with them.
We promised that this article series wouldn’t require technical understanding of AI as a prerequisite. Here’s an over-simplified explanation on how these two factors contribute to biases in AI and ML models. (If there’s interest, we’ll add a future article going into this topic in more depth.)
How AI and ML models work
They rely on probabilities to make predictions and choices among options. In general, less common options are less likely to be chosen.
Example: If option A is 60% likely and option B is 40% likely, the 60% likely option A is more likely to be selected by a prediction model based on the internal patterns found during the training phase. This can cause the 40% option B cases to not be well represented in the outputs, i.e. create a bias against the 40% B’s.
As noted above, we are oversimplifying the problem. In real life, the algorithms sometimes make associations and learn from patterns that are not equally distributed among the whole dataset because of “selection bias”. This can happen when ML algorithms can learn some patterns more easily, depending on their distribution, but unfortunately other patterns may be more difficult for the algorithm to learn. The result is that the model converges on the more frequently “seen” distribution.
How we collect & use data with AI/ML
AI and ML models depend on, and reflect, the biases and imbalances inherent in the data they were trained on. If the dataset is “unbalanced” or unrepresentative, then the results of models trained on that dataset can be unfair or un-useful. Some examples:
If 60% of people in the world are actually A, but only 25% or less of the training dataset is identifiable as A, then the results from the models are likely to be biased against A.
If the AI model works poorly on the 25% A’s it knows about, and works well on the other 75%, then the outputs of the models will not be fair to A’s.
There are machine learning design and implementation techniques which can mitigate these problems and provide good, fair results across all segments in unbalanced datasets. Like other AI technologies, they are improving in effectiveness over time. Companies and research studies have focused on decreasing and measuring the bias rates. But:
It’s still hard; it takes committed time and effort to apply them and eliminate bias.
The work needs to be ongoing. As the model interacts with fresh real-world data, patterns and balances can shift, and models can degrade in accuracy and fairness.
Even when we are trying to select the appropriate data at every stage, we can’t completely avoid biases on ML models. Real data is inherently biased, and it’s common that some data and distributions will be more abundant.
When rushing to get to market first with a new tool preview, this hard work may be de-prioritized or skipped in organizations that aren’t strongly committed (top to bottom) to ethical, responsible AI. A recent Washington Post article quoted prominent AI scientist Dr. Timnit Gebru (former leader of Google’s ethical AI team) on this concern: “To me, it feels like they’re in a race, and they just want to win the race, and anybody who’s doing anything else is useless”10.
Bias minimization is critical to ethical AI. It's also technically difficult and time-consuming - but that’s no excuse for not doing it.
These bias concerns apply to TTA and non-TTA generative AI models which are trained on songs. Using Text to Audio (TTA) as an input step introduces additional risks, since many current large language models (LLMs) for text prompting have biases and ethics issues of their own.
Using text input with LLMs as a step in generating music (or other media, such as video) with AI can be convenient for users, but it can propagate and worsen existing biases and ethical concerns in the LLMs into the generated music.
We’ll discuss bias concerns in depth in PART 6 in this series, on Bias & Diversity.
IP RIGHTS & ETHICS
Another key area of concern for ethics in generative AI for music is around ownership and protection of intellectual property rights.
Ownership of AI-assisted works
1. by music contributors
Although opinions vary somewhat by region, world laws on copyright generally recognize that the composers and performers have ownership rights in their creative works.
Musicians are increasingly speaking up to affirm that they do not accept the use of their music without licensing and permission, and the resulting devaluation of their music. Over 200 artists with the Artist Rights Alliance recently petitioned tech companies to stop doing this11.
For an excellent historical perspective on the law and music, including the Napster days and the infamous “Drake + The Weeknd” AI-based mashup that they didn’t consent to, see this article12 .
One position I’ve heard on IP rights for contributors is that in some regions, a majority of people believe it’s ok to use another person’s creation as the basis for something new. IMHO that is not sufficient reason to ignore contributors’ IP rights in the socio-technical systems we design and use for the “music economy”.
My perspective on music IP rights is pro-choice:
People who believe creative works should be free for others to reuse are welcome to release their own creations as “public domain”; they do not need any special laws or agreements to support them in doing this. But:
No one should have the right to essentially force others who do not share their belief to have their creations also treated as public domain.
“My body (of music), my choice.”
For example, musician Holly Herndon has taken the position that her “voice is for everyone”13. She should be fully entitled to make that choice. But no one should be empowered to impose that choice on other performers.
Regardless of whether some or all of a region’s creators are ok with public domain treatment of their own works, the systems we design for global treatment of contributors’ creations need to respect and enforce the protection of rights for musicians who choose not to have their works defaulted to being public domain.
These rights require that music contributors have the benefit of the “4Cs” (Consent, Control, Credit, and Compensation) regarding their creative works.14
2. by music consumers (genAI Tool Users)
Here again, opinions vary somewhat by region. There are as yet no clear legal standards on what ownership rights belong to someone who uses a genAI tool to create a ‘new’ song. This area of the law is likely to continue to evolve.
To whatever extent a tool user may have ownership rights to their new song, they should enjoy the same “pro-choice” support as music contributors over its use.
However, odds are good that most tool users don’t really understand what rights they may or may not have, even now. “Click-wrap” software licenses and Terms & Conditions of Use (T&Cs) have been notorious for decades for:
being written in legalese that the average person can’t readily understand,
being odiously long and with a paltry UX for reading,
not being transparent about what rights someone is giving up by using the software, or when the terms have changed in a meaningful way.
Accordingly, they are not read by a vast majority of users, and many of those who do scroll through the long legalese don’t truly understand them.
AI tool licenses and T&Cs are generally no different. This makes it highly likely that the average person using generative AI tools to make music doesn’t fully understand the bias and IP risks they are taking with the tools and with the works they create with them, or what rights they do & don’t have for their song.
Even law firms don’t always understand AI software T&Cs on privacy and confidentiality, as reported by journalists Isha Marathe and Cassandre Coyer in this story15 about Microsoft’s Azure OpenAI and leakage of client confidential info. How could we possibly expect that most ordinary people will understand T&Cs for genAI music tools?
Informed Consent view: From an ethics perspective, genAI tool providers need to strive to ensure that people who use their genAI tool know what rights they do & don’t have, and what risks they are taking based on how they might use a new song they’ve generated with the tool.
3. by genAI tool providers
One key question is whether our third group of stakeholders (tool provider companies and staff) has IP rights. Can the companies, or their AI-based software tools, claim ownership of works generated with the tools?
Tools: maybe? In South Africa, and then in Australia, the courts ruled that the DABUS AI-based system which created an improved design for a food container could be legally recognized as an inventor on a patent application for its food container.1617 However, courts in the US and western Europe have ruled that DABUS (and by extension, potentially any AI-based tool) is not and cannot be considered to be an ‘inventor’ or creator of a work the tool produces.1819 An “Artificial Inventor Project” has been started to track “a series of pro bono legal test cases seeking intellectual property rights for AI-generated output in the absence of a traditional human inventor or author”.20
Patent and copyright aren’t the same, though, and copyright law is more pertinent to music.21 This Billboard article22 provides a good summary of legal history and recent events in AI and music copyright through (date).
Companies: maybe? It’s worth noting that some genAI music tool providers (e.g. Boomy and Beatoven) claim and hold copyright on the works generated from their tool, as part of their business model. Their Terms and Conditions for use of their tools clearly define ownership rights for tool users and for the company. So far, the legal view that an AI-based tool cannot be an inventor doesn’t appear to impede the firms from holding copyright.
However, ethical tool providers still need to take ownership of providing enough information to their customers and tool users to ensure that they are giving informed consent to the Terms and Conditions, so they truly understand:
what ownership and usage rights they do and don’t have on their creations, and
what risks they may be taking, e.g. from potential biases in the tool or works, or by copyright infringement of contributors’ IP
Accordingly, in the following sections, we will ignore tool providers and focus on:
music contributors (creators of the music used for training AI) and
music consumers (people who use the genAI tools to create something new)
Model Training
There are two options for tool providers to address concerns about IP rights of music contributors - people whose original music is used as input for training the models:
Only using non-copyrighted (e.g. public domain) or already-owned source materials for training models.
Ensuring that proper licensing and credit are secured for any copyrighted materials before they are using for training a model, and providing fair remuneration to creators when their copyrighted materials are reused in an AI-generated creation (the “4Cs”).
Option 1 is simple and conceptually easy. However, reliance on non-copyrighted materials precludes use of most modern-day music for training models for any organization that doesn’t already own a huge dataset of source materials and isn’t wealthy enough to buy one.
This could be foreseen to constrain the musical genres which these tools can support (e.g. classical, jazz, and blues may be supportable; punk, grunge, techno, and rap might not). This limitation does not appear to be a showstopper, though.
For example, Boomy supports multiple genres (electronic dance, rap beats, lo-fi, global groove, relaxing meditation, experimental, and custom) and they state that they do not use any copyrighted material for training their models. As of March 21, 2024, artists have created over 19 million ‘original’ songs with Boomy.
As mentioned earlier, Boomy holds the copyrights on those songs, while allowing relatively free use of the songs by their creators, including for commercial purposes. Other similar tools, e.g. Beatoven, do likewise.
Option 2 opens up the pool of potential training material to all time and the whole world. However, negotiating for rights is technically and logistically complicated, and potentially expensive and time-consuming.
A number of firestorms have broken out with regard to companies using copyrighted materials for training generative AI models without licensing them. The most notable recent example is the uproar over the March 11 WSJ interview by Joanna Stern with OpenAI CTO Mira Murati. Her answer on possible scraping of YouTube videos and other public sources to train their Sora generative AI tool (text to video) was far from reassuring. We will discuss this incident more in PART 4, Training and Reference Data. If you’d like to get a head start on the topic, see the full WSJ article from March 1323.
It’s worth noting that in partial response to the ethical concerns around fair use and IP ownership, startups are arising to help companies validate that their LLMs have been trained fairly. One example is Fairly Trained, a “non-profit certifying generative AI companies for fairer training data practices”24, launched in January 2024.
Consensus has been emerging around “3Cs” and “4Cs” for protecting the rights of music contributors, whether their works are used in pretraining, training, fine tuning, or at model usage time.
Model and Song Usage & Compensation
Knowing whose contributions were used in training a music generation model is only part of the problem. A key challenge in properly crediting (and paying) music contributors is knowing whose contributions were actually used and ended up, in some way, in the generated output. This is often called ‘data provenance’.
Note that there is a corresponding techno-legal challenge in determining the criteria or thresholds for deciding whether source material was ‘used’ in a generated song. These criteria might vary widely across regions and evolve over time as case law and precedents are established.
Providing traceability to know when a specific music contributor’s work ends up in an AI-generated work is admittedly hard - but is probably not impossible. Researchers are already making progress on “computational copyright” and royalty models for compensating musicians for use of their original work in AI-based tools.25
Traceability - knowing which source pieces of music from the training data are being “reused” in a genAI creation (and to what extent), for which the original creator should be credited - is both technically challenging and critical for ensuring ethical “fair use” under Option 2.
These concerns will be explored further in PART 4 and PART 5 in this article series, on Training & Reference Data and Traceability & Fair Use.
VIEWS ON ETHICAL RISKS
Given these concerns, where do the people of the world stand on ethics principles and how they apply to generative AI?
Standards & Regulations
Where industry standards and regulations have been established, they can provide insights into what the people in those regions support and believe. Several excellent regional and international guidelines have been emerging in the past few years on ethics of artificial intelligence and how to design AI-based systems which are inherently ethical.
At this writing, we still have a global hodge-podge of AI guidelines, voluntary standards, and emerging regulations. If you’re curious about emerging AI ethics frameworks in the US and other countries, and at regional or global levels, please check out this bonus PART 2 supplemental page for details on AI Ethics Standards.
The good news is that both of the key concerns on generative AI for music, biases and IP rights, are prominent in most emerging guidelines and regulatory frameworks. In addition to respect for IP rights (including copyright), the March 21 UN resolution calls out AI for addressing “disability, gender, and racial equality”. For a quick deep dive into these equality aspects of the UN Resolution on AI, see our March 24 post A step towards global "safe, secure, and trustworthy AI”.
End Users & Ethics
This “Water and Music” article26 highlights a critical limitation of the major AI ethics frameworks: lack of focus on end users.
“One caveat of these widely available AI ethics frameworks is how they’re primarily focused on the research, development and business practices of AI. This is understandable, since the main responsibility lies with AI developers and policy-makers. But addressing ethical aspects of AI usage by end-users is essential.”
The article identifies the “Framework for Ethical Decision Making”27 by the Markkula Center for Applied Ethics as one potential approach.
Public Opinion on Ethics of Generative AI
An excellent 2023 “Generative AI & American Society Survey” by the National Opinion Research Center28 gives us three great insights29 into US residents’ opinions on ethics of generative AI in particular:
A majority now “support paying artists and creators if their work is used to train AI systems” (57% agree and only 8% disagree) - i.e., Americans are overwhelmingly “pro choice” on music contributors’ IP rights
Half “favor companies being more transparent about how AI models work and what data they use”
Two-thirds “favor labeling AI content” (content generated by AI)
Opinions in other regions may vary somewhat. (Results from similar surveys from other regions will be shared in future as they are reported.)
SUMMARY
Key Ethical Risks and Challenges
Here in PART 2, we’ve highlighted some ethical risks faced by all three stakeholder groups: music contributors, music consumers, and music tool providers. The two main risks are:
Bias minimization to prevent harm to all stakeholder groups, as well as the public
Protection of IP rights for use of songs by contributors and tool users
Both bias minimization and protection of IP rights are critical to ethical AI and technically challenging. That’s no excuse for not doing them, though.
In fact, protecting the music consumers who use these tools from the risks of generative AI for music, and ensuring the viability of these new tools and businesses, requires proactively prioritizing both bias minimization and IP protection.
Having an ethical music economy and community in the age of generative AI requires us to advocate for, and achieve, 3 things:
Pro-choice support in our socio-technical music economy for music contributors’ IP rights, including traceability to new creations
Prioritization of bias minimization efforts by tool providers
Robust support from tool providers to be transparent with music consumers (tool users) so they can have truly informed consent about their rights and the risks they may be taking, and they can make decisions consistent with their ethical principles about which tools to choose and use (or not)
What’s Next
Next up in this article series is PART 3 - a summary of the WHO, WHAT, & WHEN: the products and companies involved in genAI for music, including major companies (OpenAI, Google, Meta, Microsoft, and Adobe) and some lesser-known enterprises.
(subscribe to be notified automatically when new articles in the series are published - it’s free!)
While we’re working on finalizing PART 3 and beyond, we’d love to hear from musicians, music lovers & consumers, technical folks, and people who are two or more of those! What do you think? Did we miss any important concerns that matter to you, or do you have a different view on some points?
CREDITS & FOOTNOTES
Big thanks for their kind contributions and feedback on early drafts of this article to:
Fabio Morreale, “Where Does the Buck Stop? Ethical and Political Issues with AI in Music Creation”, 2021. Transactions of the International Society for Music Information Retrieval, 4(1), pp. 105–113. DOI: https://doi.org/10.5334/tismir.86
John Tasioulas/Ada Lovelace Institute, “The role of the arts and humanities in thinking about artificial intelligence (AI)”, June 14, 2021
Anton Vokrug/HackerNoon, “Navigating the Ethics of Artificial Intelligence”, Oct. 26, 2023
“Ethics In The Age Of AI” , Markkula Center for Applied Ethics at Santa Clara University, Nov. 2023
Brian Merchant, “The tide is turning against OpenAI”, March 15, 2024
Shayne Longpre, Robert Mahari, Naana Obeng-Marnu, William Brannon, Tobin South, Jad Kabbara, and Sandy Pentland, “Data Authenticity, Consent, and Provenance for AI Are All Broken: What Will It Take To Fix Them?”, March 27 2024
Mark Montgomery, Working paper on SPEAR AI systems (Safe, Productive, Efficient, Accurate, and Responsible)”, 2023-2024
AI Incident Database, Incident 103: Twitter’s Image Cropping Tool Allegedly Showed Gender and Racial Bias, Sept. 18, 2020
Alex Hern/The Guardian, “Twitter apologises for 'racist' image-cropping algorithm”, Sept. 21, 2020
Gerrit De Vynck and Will Oremus/Washington Post, “As AI booms, tech firms are laying off their ethicists”, March 30, 2023 (paywalled)
Artist Rights Now, “200+ Artists Urge Tech Platforms: Stop Devaluing Music“, April 2, 2024
Chris Dalla Riva/AI Supremacy, “AI and the future of music production”, March 27, 2024
Camille Sojit Pejcha/Document Journal, “Who does your voice belong to? For musician Holly Herndon, the answer is ‘everyone’”, Jan. 27, 2022
Credit for the 4Cs (consent, control, credit, compensation) phrasing goes to the Algorithmic Justice League (led by Dr. Joy Buolamwini).
Credit for the original 3Cs (consent, credit, and compensation) belongs to CIPRI (Cultural Intellectual Property Rights Initiative) for their “3Cs' Rule: Consent. Credit. Compensation©.”
Isha Marathe and Cassandre Coyer, LinkedIn post on Microsoft’s Azure OpenAI Service’s ‘abuse monitoring’ policy and its impact on law firms, March 20, 2024
Andrew H. DeVoogd/Mintz - Intellectual Property Viewpoints, “Intellectual Property — Can Our Creations Also Create? The DABUS AI System as a Named Inventor [PODCAST]”, October 13, 2021
Rebecca Currey & Jane Owen/Bird & Bird in WIPO Magazine, In the Courts: Australian Court finds AI systems can be “inventors” , Sept. 2021
Matthew Nigriny and Gary Abelev/American Bar Association, “Can an AI Be an Inventor? In the US, the Answer Remains No”, May 25, 2023
Richard M. Assmus, Brian W. Nolan, Dr. Ulrich Worm, Benjamin Beck, Mark A. Prinsley, Oliver Yaros, Reece Randall, Ondrej Hajda, Ellen Hepworth, Alasdair Maher/Maher|Brown, “Can AI Be An Inventor? The US, UK, EPO and German Approach”, Jan. 9, 2024
The Artificial Inventor Project, https://artificialinventor.com/
Congressional Research Service/US Congress, “Generative Artificial Intelligence and Copyright Law”, updated Sept. 29, 2023 (music is discussed on page 4)
James Sammataro, Nicholas Saady/Billboard, “How Much Humanity Will AI-Generated Songs Need to Be Copyrightable? (Guest Column)”, 2023-01-27
Joanna Stern/WSJ, “OpenAI Made AI Videos for Us. These Clips Are Good Enough to Freak Us Out”, article and video with OpenAI CTO Mira Murati, March 13, 2024
Junwei Deng & Jiaqi Ma/University of Illinois Urbana-Champaign, “Computational Copyright: Towards A Royalty Model for AI Music Generation Platforms”, Dec. 11, 2023 arXiv:2312.06646v1
Sarah LaReau/Water and Music, “Making music with AI? Start with these ethical guidelines”, 2023-06-08
Markkula Center for Applied Ethics at Santa Clara University, “Ethics Resources: A Framework for Ethical Decision Making”
James McCammon/96layers.ai, “Americans broadly favor Responsible AI policies”, Sept. 27, 2023
James McCammon/96layers.ai, “Majority of Americans support paying creators if their art is used to train AI models”, Sept. 25, 2023
Wow, that was a great read. But very difficult to comment on, since every angle I could think of was covered in the article.
You mentioned traceability briefly, and I look forward to your thoughts on that in subsequent articles. One idea I have been thinking about is embedding machine-readable metadata into media that could potentially be used as input to an LLM or other AI tool. That could potentially mitigate some of the IP issues you identified, by maintaining a strong mapping between source- and derived material.
Steganography is a mature technology that allows embedding of data that is imperceptible to humans but readable by machines in certain types of media, including music and graphics.
It would be helpful if there were a standard for embedding copyright-and origin metadata into media. A human author would encode: "I am Joe Bloggs, I created this work with no help from AI. I retain full copyright, but I will allow reasonable re-sampling by human composers at no charge. AI authors must pay a royalty fee for using this work. "
An AI might encode: "I am DeepThought. I created this work based on works by the following artists: [Joe BLoggs, ...]. I paid them royalty as appropriate. Any re-use of this work must credit the artists listed above".
That would level the playing field and protect everyone. It would also solve a huge problem for the AI industry. Namely, it would allow AI-generated works to be identified *by AIs* and would therefore help prevent the garbage-in-garbage-out threat presented by AIs getting trained on AI-generated works.
The tricky bit would be preserving the metadata throughout the mangling of an LLM. Ideally you want ALL the sources to be credited in the end product. I suspect that adding metadata vectors in the Attention block might be the solution.
Interesting post by Johan Cedmar-Brandstedt on artists’ rights as humans, not just their IP ownership rights. To me the 1966 agreement feels like a universal agreement on the relevant ethics, even if our laws are still a lagging global patchwork.
https://www.linkedin.com/posts/johan-cedmar-brandstedt-a77b311_artist-rights-are-human-rights-activity-7189354525999185920-g-0I